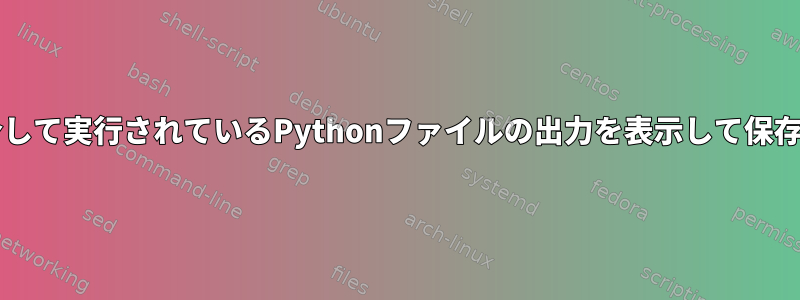
私はLinuxとプログラミングの世界に初めて触れたので、私の質問は非常に基本的ですが、どこでも答えを見つけることができません。
私はシェルファイルを使用して仮想クラスタでPythonファイルを実行しています(回帰を実行するため、テーブルを取得すると予想されます)。ジョブは正常に起動して実行されますが、結果を視覚化したり(メッセージが表示された場合は印刷)、出力を含むファイルを作成することはできません。
Pythonファイルの結果を表示するにはどうすればよいですか? BashやPythonコードに入れることができるコマンドはありますか?
python mycode.py > SomeFile.txt
SomeFile.txtという空のtxtファイルを生成するbashに次のコマンドを入力するなど、うまくいかないいくつかの解決策を試しました。
また、texファイルとpickleファイルから結果を得るためにPythonコードに次の行を追加しようとしましたが、どちらも機能しませんでした。
print(f"Output file: {results.data.htmlFileName}")
results.writeLaTeX()
print(f'LaTeX file: {results.data.latexFileName}')
results.writePickle()
print(f'Pickle file: {results.data.pickleFileName}')
どんな助けでも事前に感謝します:)
私はクラスタで実行したいPythonコードのより良いアイデアを得るためにmycode.pyのスクリプトの下に挿入しました。 (小さい注:これが私が実行する最初のコードなので、テストで5 Monteだけを要求します。Carloは引き分けですが、目標は100000引き分け以上を要求することです)
#Import packages
import pandas as pd
import biogeme.database as db
import biogeme.biogeme as bio
import biogeme.models as models
from biogeme.models import logit
from biogeme.expressions import Beta, DefineVariable, bioDraws, log, MonteCarlo
from datetime import datetime
#Prepare the data
step2_sup3_7ex = pd.read_table("step2_sup3_7ex.txt")
step2_sup3_7ex
database = db.Database("step2_sup3_7ex",step2_sup3_7ex)
# The following statement allows you to use the names of the variable
# as Python variable.
globals().update(database.variables)
#model specification
#parameters to be estimated: as a reference mode, we choose the car
#Alternative specific constant:
ASC_CAR = Beta ( "ASC_CAR" ,0 ,None ,None , 1 ) #1 as a last parameter so it will not be estimated since we decided to have the car as a reference alternative
ASC_PT = Beta ( "ASC_PT" ,0 ,None ,None , 0 )
ASC_BICYCLE = Beta ( "ASC_BICYCLE" ,0 ,None ,None , 0 )
#alternative specific attributes
#the used starting values are 0 because from exeperience with S2B3 using the starting values of prevous models gives results with low goodness of fit
B_COST = Beta("B_COST",0,None,None,0)
B_TIME_CAR = Beta("B_TIME_CAR",0,None,None,0)
B_TIME_PT = Beta("B_TIME_PT",0,None,None,0)
B_TIME_BICYCLE = Beta("B_TIME_BICYCLE",0,None,None,0)
B_POLL_mean = Beta("B_POLL_mean",0,None,None,0)
B_POLL_std = Beta("B_POLL_std",0.1,None,None,0)
B_PHYS_mean = Beta("B_PHYS_mean",0,None,None,0)
B_PHYS_std = Beta("B_PHYS_std",0.1,None,None,0)
#Individual effect of the % of people adopting a behavior
B_PRCT50 = Beta("B_PRCT50",0,None,None,1)
B_PRCT75 = Beta("B_PRCT75",0,None,None,0)
B_PRCT90 = Beta("B_PRCT90",0,None,None,0)
#we cross the level of risk of sickness due to air pollution with the % of people adopting the mobility behavior: pour chaque niveau on a un paramètre spécifique
B_POLL_PRCT50_mean = Beta("B_POLL_PRCT50_mean",0,None,None,1)
B_POLL_PRCT50_std = Beta("B_POLL_PRCT50_std",0.1,None,None,1)
B_POLL_PRCT75_mean = Beta("B_POLL_PRCT75_mean",0,None,None,0)
B_POLL_PRCT75_std = Beta("B_POLL_PRCT75_std",0.1,None,None,0)
B_POLL_PRCT90_mean = Beta("B_POLL_PRCT90_mean",0,None,None,0)
B_POLL_PRCT90_std = Beta("B_POLL_PRCT90_std",0.1,None,None,0)
#Risk variation (specific delta for each health attribute)
delta_POLL = Beta('delta_POLL',0,None,None,0) #delta is positive, varies from 0 to +infinity
delta_POLL75 = Beta('delta_POLL75',0,None,None,0)
delta_POLL90 = Beta('delta_POLL90',0,None,None,0)
delta_PHYS = Beta('delta_PHYS',0,None,None,0)
#parameters of the status quo
B_STAT_CAR = Beta("B_STAT_CAR",0,None,None,1)
B_STAT_PT = Beta("B_STAT_PT",0,None,None,0)
B_STAT_BICYCLE = Beta("B_STAT_BICYCLE",0,None,None,0)
#Socioeconomic parameters:
B_AGE = Beta('B_AGE',0,None,None,0)
B_AGE2 = Beta('B_AGE2',0,None,None,0)
B_GENDER = Beta('B_GENDER',0,None,None,0)
# Random parameters
B_PHYS_random = B_PHYS_mean + B_PHYS_std * bioDraws('B_PHYS_random','NORMAL')
B_POLL_random = B_POLL_mean + B_POLL_std * bioDraws('B_POLL_random','NORMAL')
B_POLL_PRCT50_random = B_POLL_PRCT50_mean + B_POLL_PRCT50_std * bioDraws('B_POLL_PRCT50_random', 'NORMAL')
B_POLL_PRCT75_random = B_POLL_PRCT75_mean + B_POLL_PRCT75_std * bioDraws('B_POLL_PRCT75_random', 'NORMAL')
B_POLL_PRCT90_random = B_POLL_PRCT90_mean + B_POLL_PRCT90_std * bioDraws('B_POLL_PRCT90_random', 'NORMAL')
#Utilities
U_CAR = ASC_CAR + B_TIME_CAR * temps1_C1Q + B_COST * cout1_C1Q
U_PT = (ASC_PT + B_TIME_PT * temps2_C1Q + B_COST * cout2_C1Q +
B_PHYS_random * (phys_act1_C1Q - phys_act2_C1Q)**delta_PHYS +
(B_POLL_random + B_POLL_PRCT75_random * prct75 + B_POLL_PRCT90_random * prct90 ) * (poll1_C1Q - poll2_C1Q)**(delta_POLL + delta_POLL75 * prct75 + delta_POLL90 * prct90) +
B_PRCT75 * prct75 + B_PRCT90 * prct90 +
B_STAT_PT * stat_TC + B_STAT_BICYCLE * stat_velo +
B_AGE * age_cont + B_AGE2 * age2_cont + B_GENDER * homme)
U_BICYCLE = (ASC_BICYCLE + B_TIME_BICYCLE * temps3_C1Q + B_COST * cout3_C1Q +
B_PHYS_random * (phys_act1_C1Q - phys_act3_C1Q)**delta_PHYS +
(B_POLL_random + B_POLL_PRCT75_random * prct75 + B_POLL_PRCT90_random * prct90 ) * (poll1_C1Q - poll3_C1Q)**(delta_POLL + delta_POLL75 * prct75 + delta_POLL90 * prct90) +
B_PRCT75 * prct75 + B_PRCT90 * prct90 +
B_STAT_PT * stat_TC + B_STAT_BICYCLE * stat_velo +
B_AGE * age_cont + B_AGE2 * age2_cont + B_GENDER * homme)
#associating the utility fcts with the numbering of the altrnatives
V = {5: U_CAR,
4: U_PT,
1: U_BICYCLE,
} #V is a python dictionnary
#associate the avaiability conditions with the alternatives
av = {5: 5,
4: 4,
1: 1,
} #dictionnary
# Choice model (Random coefficients/ mixed logit model)
prob = logit(V,av,choix_C1Q)
logprob = log(MonteCarlo(prob))
biogeme = bio.BIOGEME(database,logprob,numberOfDraws=5)
biogeme.modelName = "S2A3_MLdelta_5MC"
start_time = datetime.now()
# As the estimation may take a while and risk to be interrupted, we save the iterations,
# and restore them before the estimation.
fname = "__MLdelta5MC.iters"
biogeme.loadSavedIteration(filename=fname)
# Estimate the parameters.
results = biogeme.estimate(saveIterations=True, file_iterations=fname)
#read the results
print(f"Estimation time: {datetime.now() - start_time}")
# Get the results in a pandas table
pandasResults = results.getEstimatedParameters()
print(pandasResults)
print(f"Nbr of observations: {database.getNumberOfObservations()}")
print(f"LL(0) = {results.data.initLogLike:.3f}")
print(f"LL(beta) = {results.data.logLike:.3f}")
print(f"rho bar square = {results.data.rhoBarSquare:.3g}")
print(f"Output file: {results.data.htmlFileName}")
results.writeLaTeX()
print(f'LaTeX file: {results.data.latexFileName}')
results.writePickle()
print(f'Pickle file: {results.data.pickleFileName}')
答え1
役に立つには遅すぎるかもしれませんが、あなたが探しているのは- ゆう'議論。
$ man python
。 。 。
-u Force stdin, stdout and stderr to be totally unbuffered. On systems where it matters, also put stdin, stdout and stderr in binary mode. Note that there is internal buffering in xreadlines(), readlines() and file-object iterators ("for line in sys.stdin") which is not influenced by this option. To work around this, you will want to use "sys.stdin.readline()" inside a "while 1:" loop.
。 。 。
したがって、作業ファイルに次のものを入れる必要があります。
python -u mycode.py > Somefile.text
あるいは、Pythonファイル処理を使用してデータをよりよく管理することをお勧めします。しかし、何らかの理由でできない場合は、上記の方法に従ってください。